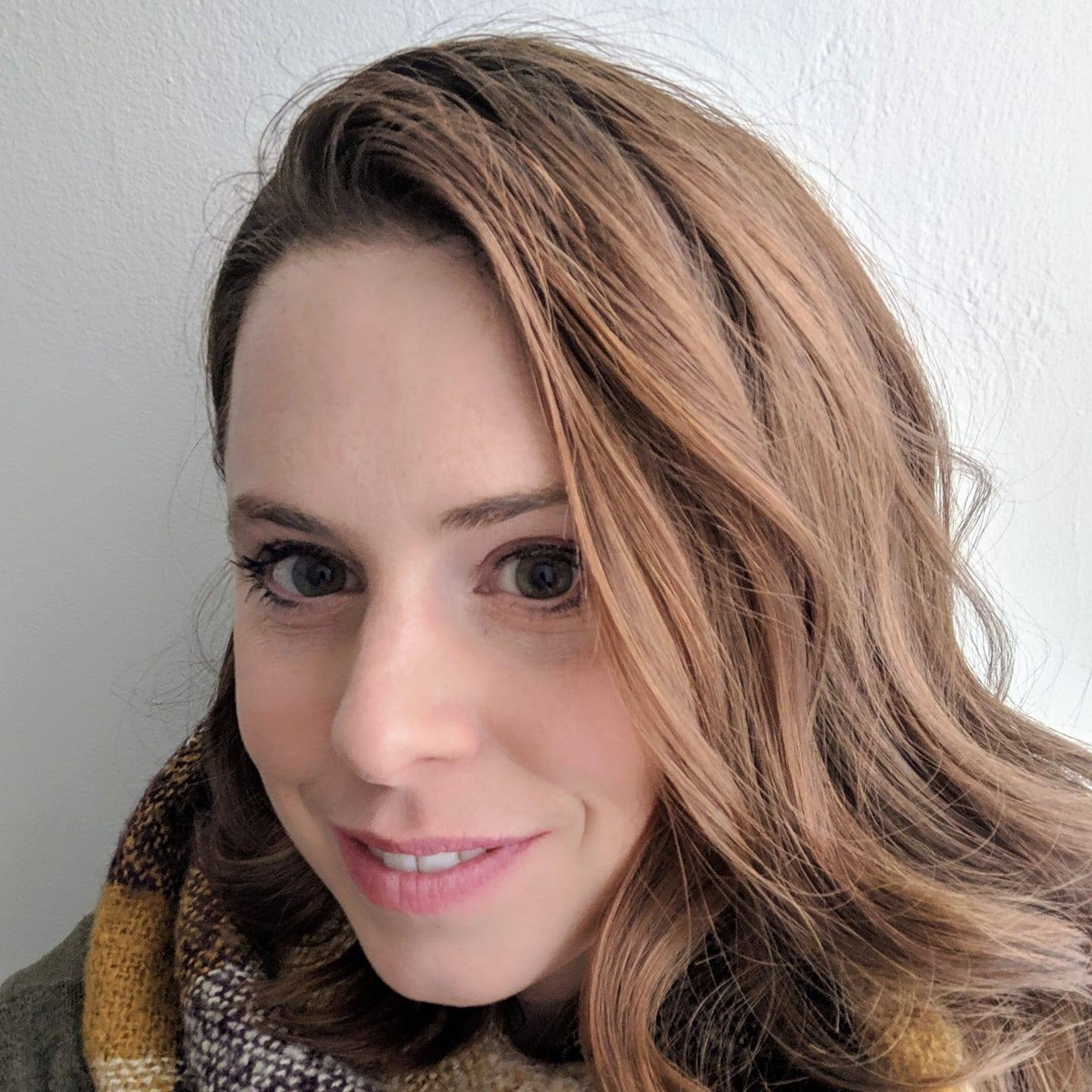
Sara Magliacane
Assistant professor
AMLab
Informatics Institute
University of Amsterdam
Lab 42, Science Park 900, 1012 WX Amsterdam
I am an assistant professor at the University Amsterdam and a Research Scientist at MIT-IBM Watson AI lab. My group focuses on three directions, causal representation learning, causality-inspired machine learning and how can causality help learn dynamical systems. Causal representation learning is the task of learning causal factors from high-dimensional data, e.g. images. Causality-inspired machine learning is the application of ideas of causal inference to machine learning, especially transfer learning and reinforcement learning. The end goal of my research is to combine these directions to make ML methods robust to distribution shift and adaptable across domains and tasks. I also continue working on my previous research on causal discovery, i.e. learning causal relations from data.
Previously I was a postdoctoral researcher at IBM Research NY, working on methods to design experiments that would allow one to learn causal relations in a sample-efficient and intervention-efficient way. I received a PhD at the VU Amsterdam on learning causal relations jointly from different experimental settings, especially in the case of latent confounders and small samples.