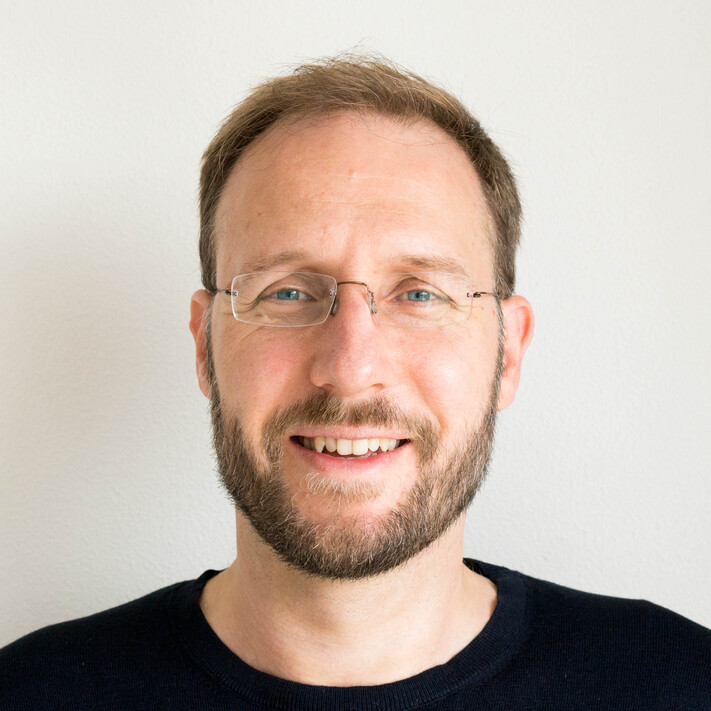
Jan-Willem van de Meent
Associate professor (UHD)
AMLab and Delta Lab
Informatics Institute
University of Amsterdam
Science Park, Lab 42, L4.13
Dr. Jan-Willem van de Meent is an Associate Professor (Universitair Hoofddocent) at the University of Amsterdam. He co-directs the AMLab with Max Welling and co-directs the Uva Bosch Delta Lab with Theo Gevers. He previously held a position as an Assistant Professor at Northeastern University, where he continues to co-advise and collaborate. Prior to becoming faculty at Northeastern, he held a postdoctoral position with Frank Wood at Oxford, as well as a postdoctoral position with Chris Wiggins and Ruben Gonzalez at Columbia University. He carried out his PhD research in biophysics at Leiden and Cambridge with Wim van Saarloos and Ray Goldstein.
Jan-Willem van de Meent’s group develops models for artificial intelligence by combining probabilistic programming and deep learning. A major theme in this work is understanding how we can develop data-efficient models in machine learning by incorporating knowledge of an underlying physical system, causal structure, or symmetries of the underlying domain. At a technical level, his group develops inference methods for probabilistic programming systems. He is one of the creators of Anglican, a probabilistic language based on Clojure and of Probabilistic Torch, a library for deep generative models that extends PyTorch. He is also an author on a forthcoming book on probabilistic programming, a draft of which is available on arXiv. To ground methodological work in practice, his group collaborates with researchers in neuroscience, NLP, healthcare, robotics, physics, and chemistry.
Jan-Willem van de Meent served as a founding co-chair of the international conference on probabilistic programming (PROBPROG). He is currently serving as a program chair for the international conference on artificial intelligence and statistics (AISTATS). He was the recipient of an NWO Rubicon Fellowship and of an NSF CAREER award.
Recent Publications
2024
-
ICLREntropy Coding of Unordered Data StructuresIn International Conference on Learning Representations (ICLR) 2024
2023
-
EMNLPCHiLL: Zero-shot Custom Interpretable Feature Extraction from Clinical Notes with Large Language ModelsIn The 2023 Conference on Empirical Methods in Natural Language Processing 2023
-
NeurIPSTopological Obstructions and How to Avoid ThemIn Thirty-seventh Conference on Neural Information Processing Systems (to appear) Dec 2023
-
CoRLOne-shot Imitation Learning via Interaction WarpingIn 7th Annual Conference on Robot Learning Nov 2023
-
ICML WNCEntropy Coding of Unordered Data StructuresIn ICML 2023 Workshop Neural Compression: From Information Theory to Applications Jul 2023
2022
-
EMNLPThat’s the Wrong Lung! Evaluating and Improving the Interpretability of Unsupervised Multimodal Encoders for Medical DataProceedings of the Conference on Empirical Methods in Natural Language Processing. Conference on Empirical Methods in Natural Language Processing Dec 2022
-
NeurIPS WSGNRUnderstanding Optimization Challenges when Encoding to Geometric StructuresIn NeurIPS 2022 Workshop on Symmetry and Geometry in Neural Representations Dec 2022
2021
-
ICML