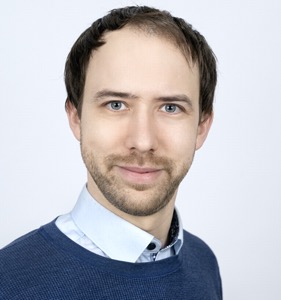
Herke van Hoof
Associate professor
AMLab
Informatics Institute
University of Amsterdam
Science Park, Lab 42, L4.05
I am associate professor at the University of Amsterdam in the Netherlands. My group works on various aspects of modular reinforcement learning. Reinforcement learning is a very general framework, but the price of that generality is generally low data-efficiency. To address that, we investigate topics like exploiting modular structures, including hierarchical structures. Such structures allowd transferring knowledge between tasks and exploiting prior knowledge, to learn more with less data. We are furthermore interested in applying reinforcement learning to domains with structured states or actions, such as learning heuristics for combinatorial problem solving.
Selected Publications
-
IJCAIValue Refinement Network (VRN)In International Joint Conference on Artificial Intelligence Jul 2022